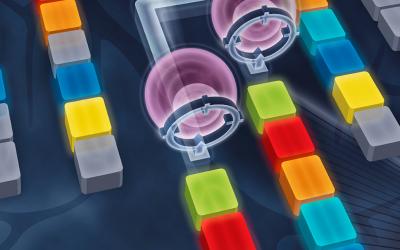
Advancing Machine Learning
Single-cell atlas projects are rapidly generating single-cell/nuclei mRNA sequencing (sc/snRNA-seq) and single-cell assay for transposase-accessible chromatin (scATAC-seq) data. My lab’s work has focused on integrating single-cell transcriptomics with genomics to assess expressed mutations (e.g. Müller et al., 2016), an important topic relevant to profiling clinical cancer specimens. This project builds on that work to address 3 significant gaps in the clinical application of single-cell ‘omics: 1) methods that integrate scRNA-seq and/or scATAC-seq with DNA sequencing (DNA-seq) to assess a clinical sample’s composition and the lineage relationships of sequenced cells; 2) algorithms to project single-cell data across reference atlases; 3) predictive models of disease progression.
Related Publications
Müller S, Cho A, Liu SJ, Lim DA, Diaz A. (2018) CONICS integrates scRNA-seq with DNA sequencing to map gene expression to tumor sub-clones. Bioinformatics 34(18):3217-3219. doi: 10.1093/bioinformatics/bty316.
Diaz AA, Qin H, Ramalho-Santos M, Song JS. (2015) HiTSelect: a comprehensive tool for high-complexity-pooled screen analysis. Nucleic Acids Res 43(3):e16. doi: 10.1093/nar/gku1197. Epub 2014 Nov 26.
Diaz A, Liu SJ, Sandoval C, Pollen A, Nowakowski TJ, Lim DA, Kriegstein A. (2016). SCell: integrated analysis of single-cell RNA-seq data. Bioinformatics 32(14):2219-20. doi: 10.1093/bioinformatics/btw201. Epub 2016 Apr 19.
Ensemble learning for classifying single-cell data and projection across reference atlases. [in preparation]